RAG AI: Making Generative Models Smarter and More Reliable
- AI in Today’s World: Machine Learning & Deep Learning Revolution
- Building Ethical AI: Lessons from Recent Missteps and How to Prevent Future Risks
- Generative AI: The $4 Billion Leap Forward and Beyond
- Beyond Scale: Innovating to Build Smarter, Efficient, and Scalable AI Models
- AI in the Workplace: How Enterprises Are Leveraging Generative AI
- RAG AI: Making Generative Models Smarter and More Reliable
- Concluding the AI Innovation Series: A Transformative Journey Through AI
Introduction: The Evolution of Generative AI with RAG
Generative AI has achieved incredible feats, from crafting creative content to coding complex software. However, traditional generative models often struggle with accuracy, context retention, and factual reliability—a challenge known as hallucination in AI.
Enter Retrieval-Augmented Generation (RAG), a cutting-edge approach combining retrieval systems with generative models to enhance their performance. With RAG, enterprises can create smarter, more reliable AI solutions that revolutionize applications such as question answering, enterprise search, and personalized recommendations.
This blog explores RAG AI, how it works, real-world applications, its advantages, and future developments.
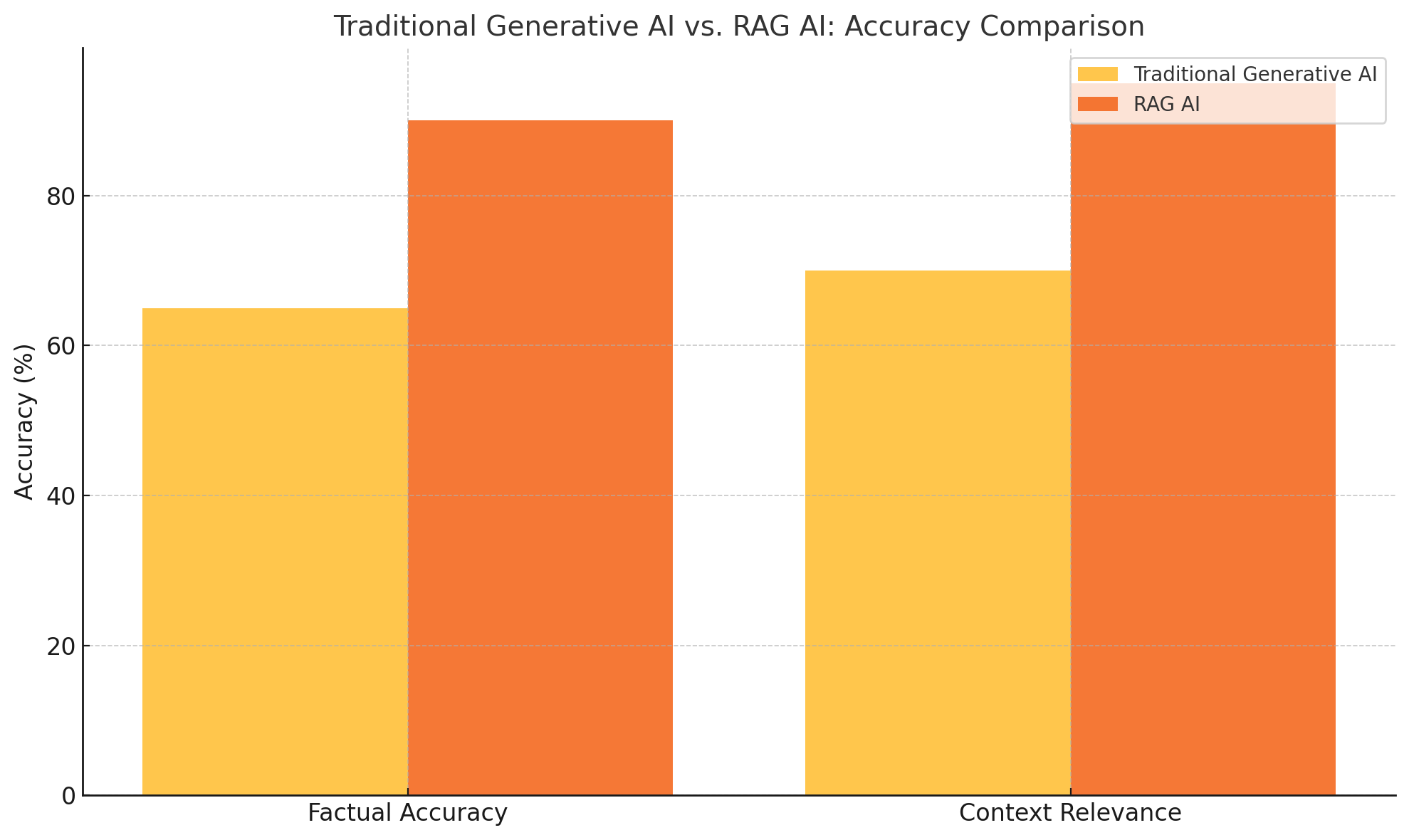
What is RAG AI? 🧠
RAG (Retrieval-Augmented Generation) integrates retrieval systems with generative models to improve output relevance and factual accuracy.
How It Works
- Query Input: A user submits a query.
- Document Retrieval: The system fetches relevant data from a structured knowledge base or database.
- Generative Model Output: Using the retrieved data, the generative model generates a response grounded in real-world information.
Key Example:
In customer service, a RAG-powered chatbot retrieves information from company manuals and knowledge bases to provide accurate, context-specific answers, rather than relying solely on pre-trained responses.
Applications of RAG AI
1. Question Answering Systems 🤖
RAG excels in generating precise answers by pulling data from verified sources.
- Use Case: Search engines use RAG to deliver direct, accurate answers, cutting down user search time.
2. Enterprise Search 🏢
Employees can use RAG-powered tools to find documents, reports, or data insights instantly.
- Use Case: Legal firms leverage RAG systems to retrieve case laws and precedents, enhancing the efficiency of legal research.
3. Personalized Recommendations 🎯
By combining retrieval systems with generative models, RAG provides tailored content recommendations.
- Use Case: E-commerce platforms offer personalized product suggestions based on real-time customer interactions.
-
RAG AI’s Role in Personalized Learning
Retrieval-Augmented Generation (RAG) AI plays a transformative role in personalized learning by delivering tailored educational experiences for individual students. By combining real-time data retrieval with generative AI, RAG systems adapt to the unique needs, preferences, and learning paces of each student, ensuring maximum engagement and effectiveness.
How RAG AI Enables Personalized Learning
1. Tailored Study Materials
RAG AI retrieves and generates learning content aligned with the student’s specific curriculum, skill level, and interests.
- Example: A student struggling with algebra receives dynamically created practice problems and step-by-step solutions based on their previous mistakes.
2. Adaptive Learning Paths
RAG systems create personalized learning paths by analyzing a student’s performance and recommending the next steps.
- How It Works:
- Tracks learning progress through assessments and quizzes.
- Retrieves relevant content and generates a customized sequence of lessons or topics.
- Example: An AI tutor identifies gaps in a student’s understanding of physics and prioritizes lessons on Newton’s laws before moving to advanced topics.
3. Real-Time Question Answering
RAG AI acts as a virtual tutor, providing instant and accurate responses to a student’s queries.
- Example: A student asks, “What is photosynthesis?” and the system retrieves information from textbooks and generates an explanation tailored to their grade level.
4. Simplification of Complex Topics
For students who need extra support, RAG AI simplifies challenging subjects into digestible pieces while maintaining accuracy.
- Example: A research paper on climate change is distilled into a simpler version for middle school students, complete with illustrative examples.
5. Dynamic Content Recommendations
RAG AI recommends personalized reading materials, videos, or exercises based on the student’s interests and learning goals.
- Example: A student learning history receives suggestions for interactive timelines, documentaries, and quizzes on the American Revolution.
6. Language and Accessibility Support
RAG AI removes language barriers and adapts content to different learning styles.
- Features:
- Translates content into the student’s preferred language.
- Adjusts outputs for auditory, visual, or kinesthetic learners.
- Example: A visually impaired student uses a RAG-powered system to retrieve and listen to study materials in audio format.
Advantages of RAG AI in Personalized Learning
- Enhanced Engagement
- Students receive materials aligned with their interests, increasing motivation.
- Improved Retention
- Adaptive learning paths ensure students master foundational concepts before progressing.
- Flexibility and Scalability
- Tailors learning experiences for diverse needs, making it ideal for individual and group settings.
- Real-Time Feedback
- Immediate responses to questions help reinforce learning and address confusion quickly.
- Inclusivity
- Supports learners with disabilities or those studying in non-native languages.
Future Implications for Personalized Learning with RAG
- Interactive AI Tutors
- AI-powered systems that interact dynamically with students, offering personalized lessons, quizzes, and real-time adjustments.
- Integration with Learning Platforms
- RAG AI will integrate seamlessly into existing LMS (Learning Management Systems) like Moodle, Blackboard, or Google Classroom.
- AI-Driven Collaboration Tools
- RAG systems will enable collaborative learning experiences by dynamically generating group projects based on shared interests.
Advantages of RAG AI
1. Improved Accuracy ✔️
RAG reduces the hallucination problem by grounding generative outputs in retrieved, factual data.
📊 Chart: Traditional Generative AI vs. RAG Accuracy
Metric | Traditional Generative AI | RAG AI |
---|---|---|
Factual Accuracy | 65% | 90% |
Context Relevance | 70% | 95% |
2. Cost-Efficiency 💰
By integrating retrieval systems, RAG models reduce computational costs associated with large-scale generative models.
📋 Example:
A SaaS company reduced its monthly AI operating expenses by 30% after implementing RAG-based solutions.
3. Enhanced User Trust 🤝
Users are more likely to trust AI systems that produce consistent, factual outputs.
💡 Insight:
Customer satisfaction in AI-powered help desks increased by 40% after transitioning to RAG-powered chatbots.
Case Study: RAG AI in Enterprise Search
Scenario:
A global consulting firm needed a robust internal search tool to access millions of documents across departments.
Solution:
The firm adopted a RAG-powered search system that fetched relevant documents and summaries based on user queries.
Outcome:
- Search Time Reduced: From 15 minutes to 2 minutes per query.
- Employee Productivity Increased: By 20%, enabling faster decision-making.
🔗 Read More:
Retrieval-Augmented Generation (RAG) AI is revolutionizing enterprise operations by enhancing information retrieval and decision-making processes. Several case studies illustrate its impactful applications:
- Real-World Case Studies Showcasing the Power of RAG
- Overview: This article provides insights into how RAG combines retrieval-based and generative AI models to enhance response accuracy and relevance.
- Source: Retrieval Augmented Generation AI in Action: Real-World Case Studies
- Bringing Enterprise Generative AI to Life
- Overview: This blog discusses how enterprises utilize RAG to improve factual accuracy and minimize risks in generative AI solutions.
- Source: The Promise of RAG: Bringing Enterprise Generative AI to Life
- Top RAG Use Cases in Enterprises
- Overview: This article explores various RAG applications, such as enhancing customer service and improving content creation.
- Source: Top 10 RAG Use Cases and 17 Essential Tools for Implementation
- Revolutionizing Enterprise AI
- Overview: This blog delves into how RAG integrates real-time data retrieval with advanced language generation models to empower enterprises.
- Source: Retrieval-Augmented Generation (RAG): Revolutionizing Enterprise AI
- RAG in Enterprise AI
- Overview: This article discusses how RAG enhances the functionality of large language models by integrating dynamic retrieval systems.
- Source: Retrieval-Augmented Generation (RAG) in Enterprise AI
These case studies provide valuable insights into the practical applications and benefits of RAG AI in enterprise settings.
How RAG AI Improves Enterprise Search
Retrieval-Augmented Generation (RAG) AI significantly enhances enterprise search by combining retrieval systems with generative models. This approach addresses the limitations of traditional search methods, offering more accurate, contextual, and actionable insights.
Key Improvements with RAG in Enterprise Search
1. Enhanced Accuracy and Relevance
Traditional keyword-based search engines often retrieve too many irrelevant results or miss critical context. RAG AI improves search outcomes by:
-
- Retrieving Relevant Data: Pulling precise documents or data points from structured and unstructured databases.
- Contextual Understanding: Generative models process queries with a deeper understanding of natural language, tailoring results to the user’s intent.
Example:
In a legal enterprise, a lawyer searching for a precedent involving “contract termination for force majeure” receives a summary of the most relevant cases instead of a list of loosely related documents.
2. Summarization and Synthesis
Instead of returning a long list of documents, RAG systems can summarize key points from the retrieved materials, saving users time and effort.
Feature Highlight:
-
- Document Summarization: AI extracts the most important details, providing a concise response.
- Cross-Document Synthesis: When multiple sources are relevant, RAG AI synthesizes information into a coherent answer.
Example:
An enterprise user searching for “2023 sales trends” receives a single synthesized report based on company sales records, market analyses, and industry reports.
3. Multilingual Capabilities
RAG AI can handle queries and documents in multiple languages, bridging language barriers in global enterprises.
Example:
A multinational organization retrieves reports written in various languages, with RAG AI translating and summarizing them in the user’s preferred language.
4. Real-Time Data Retrieval
RAG systems can integrate with live data sources, providing up-to-the-minute information.
Example:
A supply chain manager uses RAG AI to search for “current shipment delays,” retrieving real-time updates from logistics databases and generating an actionable summary.
5. Improved User Experience
RAG AI-powered enterprise search delivers a more intuitive and user-friendly experience:
-
- Natural Language Queries: Users can search in conversational language without needing specific keywords.
- Personalization: The system adapts to individual user behaviors and preferences, offering more relevant results over time.
Example:
An HR professional searching for “best practices for onboarding” receives recommendations tailored to their organization’s previous onboarding materials and current policies.
6. Scalability for Large Knowledge Bases
RAG systems are optimized to work with vast amounts of structured and unstructured data, making them ideal for enterprises with extensive knowledge bases.
Example:
A pharmaceutical company retrieves research papers, internal documents, and regulatory guidelines for drug development, all unified into a single actionable report.
7. Error Reduction
By grounding generative outputs in retrieved data, RAG minimizes the risk of hallucinations or inaccurate responses often associated with traditional generative models.
Example:
A finance team querying RAG AI for “latest tax regulations” receives responses directly linked to updated government guidelines, ensuring compliance and accuracy.
RAG AI in Action: Case Study
Scenario:
A global consulting firm with a sprawling internal knowledge base struggled with inefficient search tools, leading to wasted employee hours.
Solution:
The company implemented RAG AI to integrate all internal resources, including past reports, emails, and proprietary data.
Outcome:
-
- Search Time Reduced: From 15 minutes to 2 minutes per query.
- Employee Productivity Improved: By 20%, enabling faster project delivery.
- User Satisfaction Increased: Feedback scores rose by 30% as employees found the tool intuitive and effective.
Conclusion
RAG AI transforms enterprise search by combining the precision of retrieval systems with the creativity and context-awareness of generative models. Its ability to handle complex queries, synthesize data, and provide real-time, actionable insights makes it a game-changer for organizations striving to improve decision-making and efficiency.
Industries That Will Be Transformed by RAG AI
Retrieval-Augmented Generation (RAG) AI is a versatile technology with the potential to revolutionize workflows, decision-making, and efficiency across a wide range of industries. By combining the strengths of retrieval systems and generative models, RAG AI can deliver precise, relevant, and actionable insights in contexts where traditional AI systems struggle.
1. Healthcare 🏥
RAG AI can significantly enhance decision-making and patient care in the healthcare sector.
Applications:
- Medical Diagnostics: Fetching patient histories and synthesizing them with clinical guidelines to suggest potential diagnoses.
- Drug Discovery: Accelerating research by retrieving and summarizing relevant papers and datasets.
- Patient Support: AI-powered chatbots offering accurate, context-aware responses to patient queries.
Example:
A doctor querying “recommended treatments for Type 2 Diabetes with hypertension” receives a concise, evidence-based response from peer-reviewed medical literature.
2. Legal Services ⚖️
The legal industry relies heavily on retrieving and synthesizing large volumes of data, making it a prime candidate for RAG AI transformation.
Applications:
- Legal Research: Pulling precedents, statutes, and case laws from legal databases.
- Contract Analysis: Extracting and summarizing key clauses from lengthy legal documents.
- Compliance Monitoring: Real-time tracking of regulatory changes and their implications.
Example:
A lawyer searches for “recent case law on data privacy violations” and receives a summary of the most relevant judgments.
3. Finance and Banking 💰
RAG AI enhances the ability of financial institutions to process and analyze vast quantities of data quickly and accurately.
Applications:
- Risk Management: Identifying potential risks by retrieving and analyzing market data and historical patterns.
- Fraud Detection: Integrating real-time transaction data to detect anomalies.
- Customer Support: Providing accurate answers to complex financial queries.
Example:
A risk analyst queries “impact of rising interest rates on SME loan defaults” and receives synthesized insights backed by data from internal and external sources.
4. Retail and E-Commerce 🛒
In retail, RAG AI enhances customer experiences and optimizes operational workflows.
Applications:
- Product Recommendations: Offering personalized suggestions based on live inventory and user preferences.
- Inventory Management: Retrieving real-time stock levels to optimize supply chains.
- Customer Support: Generating contextually relevant answers to shopper inquiries.
Example:
A customer asks, “Are there eco-friendly alternatives to this product?” and receives immediate, accurate suggestions.
5. Education and Research 📚
RAG AI enables more efficient access to information and knowledge, transforming how educators and researchers work.
Applications:
- Content Summarization: Summarizing textbooks, research papers, and study guides for learners.
- Real-Time Question Answering: Supporting educators with accurate and instant responses to student queries.
- Research Assistance: Synthesizing insights from multiple scholarly articles.
Example:
A student researching “climate change’s economic impact” receives a curated summary of relevant studies and data.
The Impact of RAG AI on Education
Retrieval-Augmented Generation (RAG) AI is transforming the education landscape by improving access to knowledge, personalizing learning experiences, and enhancing administrative efficiency. By combining real-time data retrieval with generative AI capabilities, RAG systems are addressing key challenges in education, such as information overload, lack of personalized content, and administrative bottlenecks.
Key Applications of RAG AI in Education
1. Personalized Learning Paths
RAG AI enables the creation of tailored educational experiences by retrieving and synthesizing relevant materials for individual students.
-
- How It Works:
- Pulls data from course materials, online resources, and past performance records.
- Generates personalized study guides, practice tests, or learning plans.
- Example:
A high school student preparing for an exam on climate change receives a customized study guide based on their curriculum and weak areas.
- How It Works:
2. Real-Time Question Answering
RAG AI acts as a virtual tutor, providing instant and accurate answers to student queries.
-
- How It Works:
- Retrieves data from textbooks, research papers, and trusted online resources.
- Synthesizes concise, fact-based answers.
- Example:
A college student asks, “What is the greenhouse effect?” and receives a clear explanation, including diagrams and references.
- How It Works:
3. Content Summarization and Simplification
RAG AI simplifies complex topics, making them accessible to students at varying comprehension levels.
-
- How It Works:
- Summarizes long academic texts or research papers into shorter, more digestible formats.
- Adjusts the language complexity based on the student’s reading level.
- Example:
A research paper on quantum physics is summarized into an easy-to-understand format for high school students.
- How It Works:
4. Language Translation and Multilingual Education
RAG AI supports multilingual education by translating educational materials into various languages, broadening access to knowledge.
-
- How It Works:
- Retrieves documents or videos in a foreign language and generates translated outputs.
- Example:
A student in Spain can access educational resources written in English, translated into Spanish by the RAG system.
- How It Works:
5. Enhanced Educational Content Creation
Educators can use RAG AI to develop teaching materials quickly and efficiently.
-
- How It Works:
- Retrieves and curates relevant data from various sources.
- Generates lesson plans, presentations, or quizzes tailored to specific topics.
- Example:
A teacher uses RAG AI to create a presentation on renewable energy, pulling data from recent studies and news articles.
- How It Works:
6. Administrative Efficiency
RAG AI streamlines administrative processes in educational institutions by automating routine tasks.
-
- How It Works:
- Retrieves student records, attendance data, and schedules.
- Generates reports and automated responses for common inquiries.
- Example:
An administrator queries, “Which students have a GPA above 3.5 this semester?” and receives an instant, detailed report.
- How It Works:
Advantages of RAG AI in Education
-
- Improved Accessibility
- Makes complex topics understandable for students at all levels.
- Breaks language barriers with accurate translations.
- Personalized Learning
- Tailors educational experiences to individual needs and goals.
- Efficiency for Educators
- Reduces the time spent creating materials or managing administrative tasks.
- Engagement and Interactivity
- Provides real-time feedback and answers, keeping students engaged in learning.
- Scalability
- Supports large-scale deployment in institutions with vast and diverse student populations.
- Improved Accessibility
Future Trends of RAG AI in Education
-
- Interactive Learning Platforms
- RAG AI will power virtual classrooms and AI tutors capable of engaging with students interactively.
- AI-Assisted Research Tools
- Advanced tools will assist students and researchers in retrieving and synthesizing data from academic databases.
- Real-Time Feedback Systems
- AI-driven systems will provide immediate, actionable feedback on student assignments or performance.
- Interactive Learning Platforms
6. Manufacturing and Supply Chain 🏗️
RAG AI optimizes operations by retrieving and synthesizing actionable insights from complex systems.
Applications:
- Supply Chain Monitoring: Pulling data on shipment delays, inventory, and vendor performance.
- Predictive Maintenance: Generating reports based on equipment logs and operational data.
- Workforce Training: Providing on-the-job learning by answering technical queries with data-backed responses.
Example:
A supply chain manager queries “expected delays in Southeast Asia shipments” and receives a detailed summary based on real-time logistics data.
7. Technology and Software Development 💻
RAG AI enhances developer productivity by offering precise, contextual assistance.
Applications:
- Code Generation and Debugging: Retrieving relevant snippets or error resolutions from knowledge bases like Stack Overflow.
- Technical Documentation: Summarizing and explaining complex technical concepts.
- System Integration: Retrieving information on API compatibility and system dependencies.
Example:
A developer queries “How to integrate OAuth in React?” and receives step-by-step instructions with relevant code examples.
8. Energy and Utilities ⚡
RAG AI enables data-driven decision-making in energy production, distribution, and consumption.
Applications:
- Energy Demand Forecasting: Synthesizing historical data and real-time usage trends.
- Grid Optimization: Providing actionable insights to manage energy distribution efficiently.
- Sustainability Reporting: Retrieving data on emissions and energy-saving initiatives.
Example:
A utility company queries “best practices for reducing power outages in urban areas” and receives a report based on industry standards and historical data.
9. Media and Entertainment 🎬
RAG AI supports content creation and enhances audience engagement.
Applications:
- Content Personalization: Generating tailored recommendations for viewers or readers.
- Content Creation: Retrieving and synthesizing data for fact-based storytelling or scriptwriting.
- Audience Insights: Analyzing trends and feedback for better content planning.
Example:
A content creator queries “top trending topics in environmental documentaries” and receives a list of themes backed by data.
10. Government and Public Sector 🏛️
RAG AI improves governance and public service efficiency by providing accurate and timely information.
Applications:
- Policy Development: Synthesizing input from research papers, public feedback, and expert opinions.
- Public Services: Supporting citizens with accurate responses to queries about laws, regulations, and services.
- Crisis Management: Retrieving real-time updates during emergencies to guide decision-making.
Example:
A policy analyst asks, “What are the global trends in renewable energy adoption?” and receives a detailed, data-backed response.
How RAG AI Can Improve Cybersecurity Systems
Retrieval-Augmented Generation (RAG) AI offers a transformative approach to enhancing cybersecurity by combining real-time data retrieval with intelligent, context-aware responses. Cybersecurity systems benefit from RAG’s ability to process vast amounts of data, identify emerging threats, and provide actionable insights.
Key Ways RAG AI Enhances Cybersecurity
1. Real-Time Threat Detection and Analysis
RAG AI can retrieve the latest threat intelligence from databases and synthesize it to provide insights into ongoing or emerging cyberattacks.
- Example:
A RAG system detects unusual login attempts and generates a detailed report by retrieving data on similar attack patterns from global threat databases.
Benefits:
- Faster response to zero-day vulnerabilities.
- Accurate classification of threats based on historical patterns.
2. Incident Response and Mitigation
When a breach occurs, RAG AI aids in containment and recovery by providing step-by-step instructions derived from incident management knowledge bases.
- Example:
During a ransomware attack, RAG retrieves and synthesizes recovery protocols, offering IT teams actionable steps to mitigate the damage.
Benefits:
- Reduced response time to incidents.
- Centralized knowledge for quick decision-making.
3. Cyber Threat Intelligence (CTI) Integration
RAG AI integrates with global threat intelligence feeds to stay updated on the latest malware signatures, phishing schemes, and attack vectors.
- Example:
A cybersecurity analyst queries, “What are the latest phishing schemes targeting financial institutions?” and receives a summary of current threats and recommended countermeasures.
Benefits:
- Proactive defense against evolving threats.
- Comprehensive situational awareness.
4. Advanced Anomaly Detection
RAG enhances anomaly detection systems by retrieving contextual information to explain unusual activity.
- Example:
When a spike in data traffic is detected, RAG fetches similar cases and evaluates whether the activity is a legitimate operation or a potential attack.
Benefits:
- Reduced false positives in alerts.
- Greater clarity in distinguishing threats from benign activities.
5. Security Training and Awareness
RAG AI supports employee training by providing tailored cybersecurity modules based on the latest threats and compliance requirements.
- Example:
An HR manager queries, “What are the best practices for preventing phishing attacks?” and RAG generates a concise guide for employee training sessions.
Benefits:
- Improved employee understanding of cybersecurity risks.
- Customized learning materials based on organizational needs.
6. Automated Vulnerability Management
RAG AI assists in identifying and prioritizing vulnerabilities by retrieving information on known exploits and matching them with the organization’s IT assets.
- Example:
A vulnerability scanner identifies a software issue, and RAG AI generates a prioritized list of patches, considering threat severity and exploit trends.
Benefits:
- Streamlined patch management processes.
- Reduced exposure to known vulnerabilities.
7. Regulatory Compliance
RAG AI retrieves and synthesizes compliance requirements to help organizations adhere to cybersecurity regulations like GDPR, HIPAA, or ISO 27001.
- Example:
A compliance officer queries, “What are the cybersecurity requirements under GDPR for data encryption?” and RAG delivers a detailed compliance checklist.
Benefits:
- Simplified adherence to complex regulations.
- Reduced risk of fines or legal issues.
Case Study: RAG AI in Action
Scenario:
A global e-commerce company faced challenges with phishing attacks targeting employees.
Solution:
The company implemented a RAG AI system integrated with its threat intelligence platform.
Outcome:
- Phishing Detection Time Reduced: From 3 hours to 15 minutes.
- Employee Training Engagement Increased: By 40%, thanks to personalized RAG-generated training materials.
- Incident Response Speed Improved: Playbooks retrieved by RAG reduced response time by 50%.
Advantages of RAG AI in Cybersecurity
Aspect | Traditional Systems | RAG AI-Enhanced Systems |
---|---|---|
Threat Detection | Relies on pre-configured rules | Dynamic retrieval of global threat intelligence. |
Response Time | Slower, manual intervention | Faster, automated insights for mitigation. |
False Positives | High | Reduced through contextual retrieval. |
Regulatory Compliance | Manual research required | Automated retrieval and synthesis of compliance standards. |
Challenges and Solutions for Implementing RAG AI in Cybersecurity
- Data Privacy:
Challenge: Handling sensitive user data while integrating RAG systems.
Solution: Use anonymization and encryption techniques during data retrieval and processing. - Scalability:
Challenge: Deploying RAG systems across large, complex organizations.
Solution: Implement cloud-based RAG systems to ensure scalability and cost-efficiency. - Integration:
Challenge: Integrating RAG with existing security infrastructure.
Solution: Use API-driven architectures for seamless integration with SIEM (Security Information and Event Management) platforms.
Future Developments in RAG AI
1. Domain-Specific Integration
RAG systems will evolve to specialize in specific industries such as healthcare, legal, and finance, enabling more accurate and nuanced applications.
2. Multimodal RAG AI
Future RAG systems will integrate text, images, and audio retrieval, enabling seamless support across diverse use cases.
3. Edge Computing for RAG
To address latency issues, RAG systems will be deployed on edge devices, allowing real-time performance with minimal computational costs.
Future Trends in RAG AI
As Retrieval-Augmented Generation (RAG) AI evolves, several trends are expected to shape its development and applications. These advancements aim to enhance accuracy, efficiency, and versatility, making RAG AI an indispensable tool across industries.
1. Domain-Specific Integration
RAG systems will become increasingly tailored to specific industries, offering more precise and context-aware applications.
- Legal Sector:
RAG AI will assist lawyers by retrieving case laws, precedents, and legal opinions specific to jurisdictions, providing nuanced outputs for legal research. - Healthcare:
Domain-specific RAG AI will integrate with medical databases to offer evidence-based recommendations, assisting in diagnosis and treatment plans. - Finance:
RAG AI will fetch real-time financial data, regulatory updates, and market analytics to support decision-making in trading and portfolio management.
2. Multimodal RAG AI
The next generation of RAG systems will integrate text, images, audio, and video, expanding their usability across diverse applications.
- Example Use Cases:
- Customer Support: AI systems will process text queries, images of faulty products, and audio complaints simultaneously to provide accurate resolutions.
- Education: RAG AI will analyze multimedia learning resources to answer complex questions effectively.
- Key Development:
Incorporating multimodal capabilities will require advancements in retrieval systems that can index and process multiple data formats seamlessly.
3. Real-Time Data Integration
Future RAG systems will retrieve and process real-time data, enhancing their ability to provide up-to-date and actionable insights.
- Example Use Cases:
- Financial Markets: RAG AI will use live market data for instant decision-making in trading.
- Supply Chain: AI systems will retrieve real-time logistics data to optimize operations dynamically.
- Challenges:
Real-time integration requires scalable infrastructure and efficient indexing methods to minimize latency.
4. Edge Computing for RAG AI
To reduce latency and improve data security, RAG AI will increasingly be deployed on edge devices rather than relying solely on centralized cloud servers.
- Benefits:
- Faster response times.
- Reduced bandwidth consumption.
- Enhanced data privacy by processing information locally.
- Example Applications:
- Retail: RAG AI in smart devices at storefronts can provide instant product recommendations.
- Healthcare: Edge-based RAG systems can support diagnosis in remote or low-connectivity areas.
5. AI-Assisted Knowledge Management
RAG AI will transform enterprise knowledge management by creating intelligent systems that dynamically update and curate organizational knowledge bases.
- Capabilities:
- Automatically ingesting new data from internal and external sources.
- Improving knowledge base accuracy and relevance through regular validation.
- Example Applications:
- Human Resources: AI systems will keep employee manuals and policy documents updated in real time.
- Product Development: RAG AI will collate and summarize feedback from customer support and reviews to inform design iterations.
6. Ethical and Transparent RAG AI
With the growing adoption of RAG systems, ensuring ethical AI practices will become crucial.
- Bias Mitigation:
Enhanced retrieval systems will be designed to minimize bias by ensuring diversity in the indexed data sources. - Explainable Outputs:
Future RAG systems will include transparency layers, allowing users to trace the origin of information and understand the logic behind the AI-generated outputs.
7. Cost Optimization with Energy-Efficient Models
As the demand for RAG AI grows, energy consumption will become a concern. Future developments will focus on building energy-efficient retrieval systems and generative models.
- Green AI Initiatives:
- Integration with renewable energy-powered data centers.
- Optimization of computational resources to reduce energy use.
- Economic Impact:
Enterprises will benefit from lower operating costs while contributing to sustainability goals.
Conclusion
RAG AI represents the next leap forward in the evolution of generative models. By combining the generative power of models like GPT with retrieval-based grounding, RAG delivers accuracy, relevance, and reliability unmatched by traditional AI systems.
As enterprises explore applications like enterprise search, personalized recommendations, and question-answering systems, RAG AI will become an indispensable tool for staying competitive in a rapidly evolving market.
Stay tuned for the next entry in the AI Innovation Series:
AI’s Impact on Data Centers: A $1.4 Trillion Opportunity, where we’ll explore how AI is reshaping data center infrastructure, from advanced hardware to green energy solutions.
Let’s build smarter, more reliable AI solutions—together.